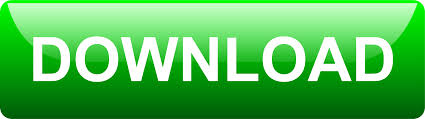
"tax" "ptratio" "black" "lstat" "medv" Split the sample data and make the model The problem statement is to predict ‘medv’ based on the set of input features.
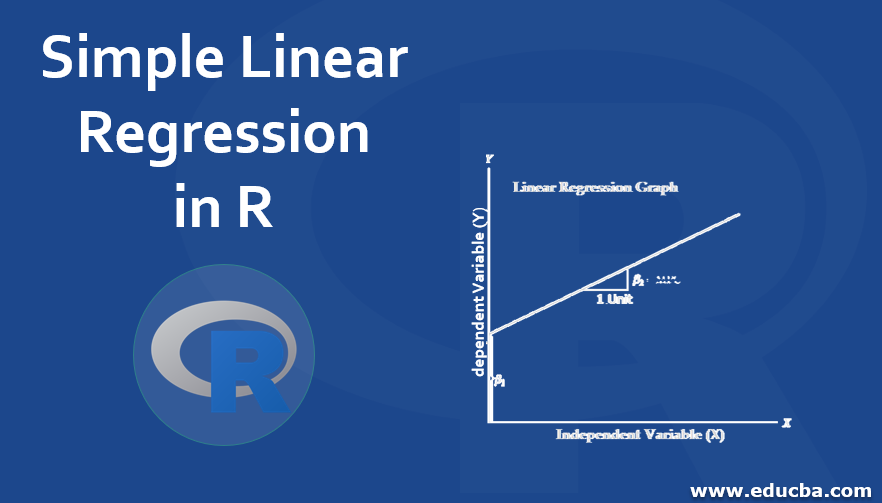
Following are the features available in Boston dataset. Extract the data and create the training and testing sampleįor the current model, let’s take the Boston dataset that is part of the MASS library in R Studio. The idea is that a particular sample may overestimate or underestimate but if one takes multiple samples and try to estimate the coefficient multiple times, then the average of co-efficient from multiple samples will be spot on. This assumption makes sure that the sample does not necessarily always overestimate or underestimate the coefficients. The basic assumption here is that the sample is not biased. The coefficient for linear regression is calculated based on the sample data.
LINEAR REGRESSION RSTUDIO HOW TO
+ βp Xp + ε ( for multiple regression ) How to apply linear regression Next step will be to find the coefficients (β0, β1.) for below model.
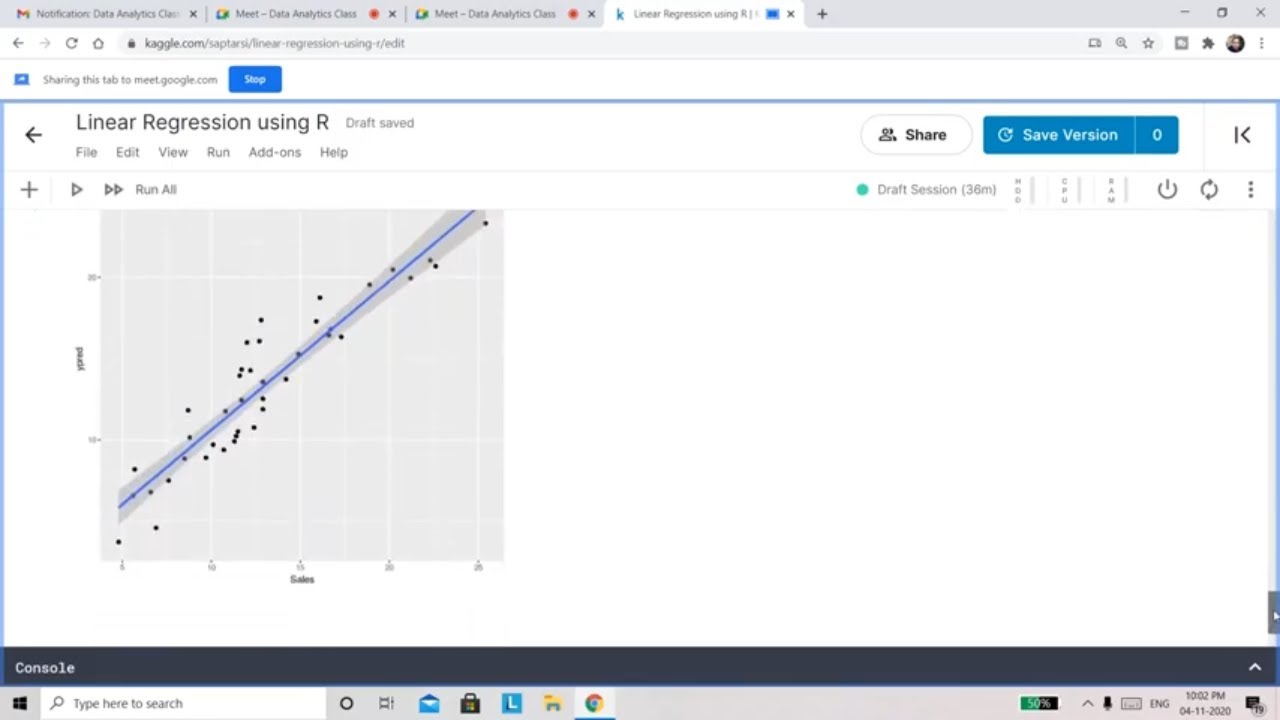
In linear regression, we assume that functional form, F(X) is linear and hence we can write the equation as below. For ideal model, this should be random and should not be dependent on any input. F(X) – defines the function that is dependent on set of input features. Let’s analyze the basic equation for any supervised learning algorithm Y = F(x) + ε The above equation has three terms: Y – define the response variable. Please note that if the basic assumption about the linearity of the model is away from reality then there is bound to have an error (bias towards linearity) in the model however best one will try to fit the model. The basic assumption here is that functional form is the line and it is possible to fit the line that will be closest to all observation in the dataset. The model fits a line that is closest to all observation in the dataset. Linear Regression is a supervised modeling technique for continuous data. In the following topic, I will discuss linear regression that is an example of supervised learning technique. Unsupervised learning does not have any response variable and it explores the association and interaction between input features.
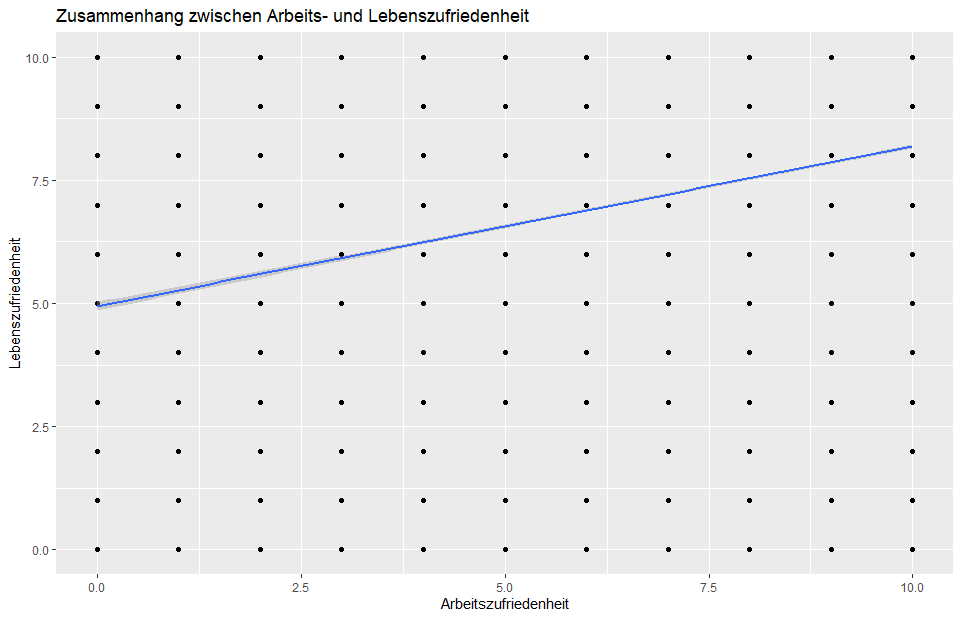
Supervised learning technique generates a response based on the set of input features. Machine learning has two distinct field of study – supervised learning and unsupervised learning. – Dashboard creation & deployment of the model. – Exploring, Cleaning and transforming data. – Defining problem statement – Data collection. ML is not only about analytics modeling but it is end-to-end modeling that broadly involves following steps: Machine Learning (ML) is a field of study that provides the capability to a Machine to understand data and to learn from the data.
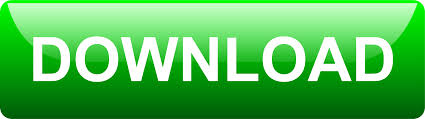